Data formats#
Data come in many forms and formats, depending on source and function.
“Flat” text files#
.txt, .dat, .csv, .fasta, …
Often with a fixed format
Comma separated columns
Tabulator separated columns
Fixed width columns
Headers, subheaders
Sections with keywords
import pandas as pd
planets_DF = pd.read_csv('../../data/planets.csv')
planets_DF.head()
planet | distance | diameter | |
---|---|---|---|
0 | Mercury | 0.387 AU | 4878 km |
1 | Venus | 0.723 AU | 12104 km |
2 | Earth | 1.000 AU | 12756 km |
3 | Mars | 1.524 AU | 6787 km |
4 | Jupiter | 5.203 AU | 142796 km |
# Using planets_DF as input, remove " AU" from the "distance" column and convert it to a float
planets_DF['distance'] = planets_DF['distance'].str.replace(' AU', '').astype(float)
print(planets_DF.dtypes)
planets_DF.head()
planet object
distance float64
diameter object
dtype: object
planet | distance | diameter | |
---|---|---|---|
0 | Mercury | 0.387 | 4878 km |
1 | Venus | 0.723 | 12104 km |
2 | Earth | 1.000 | 12756 km |
3 | Mars | 1.524 | 6787 km |
4 | Jupiter | 5.203 | 142796 km |
Plotting directly with Pandas#
Instead of converting data to NumPy arrays and plotting, it is possible to plot directly from Pandas with special plot commands and .plot.xxx().
# Bar plot
# pd.options.plotting.backend = "matplotlib"
import matplotlib.pyplot as plt
planets_DF.plot.bar(x='planet', y='distance', rot=0)
plt.show()
# Try: color, rot, add more with plt.xxx()
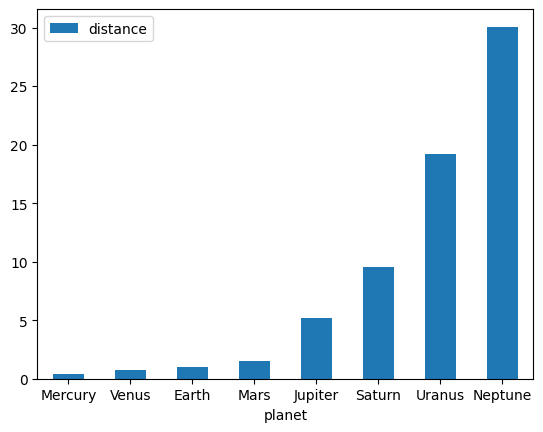
Exercise#
Plot the planets as circles instead of bars.
Use the diameter of the planet to adjust the circle size.
# Plot concentric ellipses for each planet where the size of the ellipse is proportional to
# the planet's distance. Add planet names to the plot. Let the xlim and ylim extend from -31 to 31.
import matplotlib.pyplot as plt
import numpy as np
fig, ax = plt.subplots(figsize=(10,10))
ax.set_xlim(-31, 31)
ax.set_ylim(-31, 31)
ax.set_aspect('equal')
ax.set_facecolor('black')
for i in range(len(planets_DF)):
ax.add_artist(plt.Circle((0,0), planets_DF['distance'][i], color='white', fill=False))
ax.text(planets_DF['distance'][i], 0, planets_DF['planet'][i], color='white', ha='center', va='center')
plt.show()
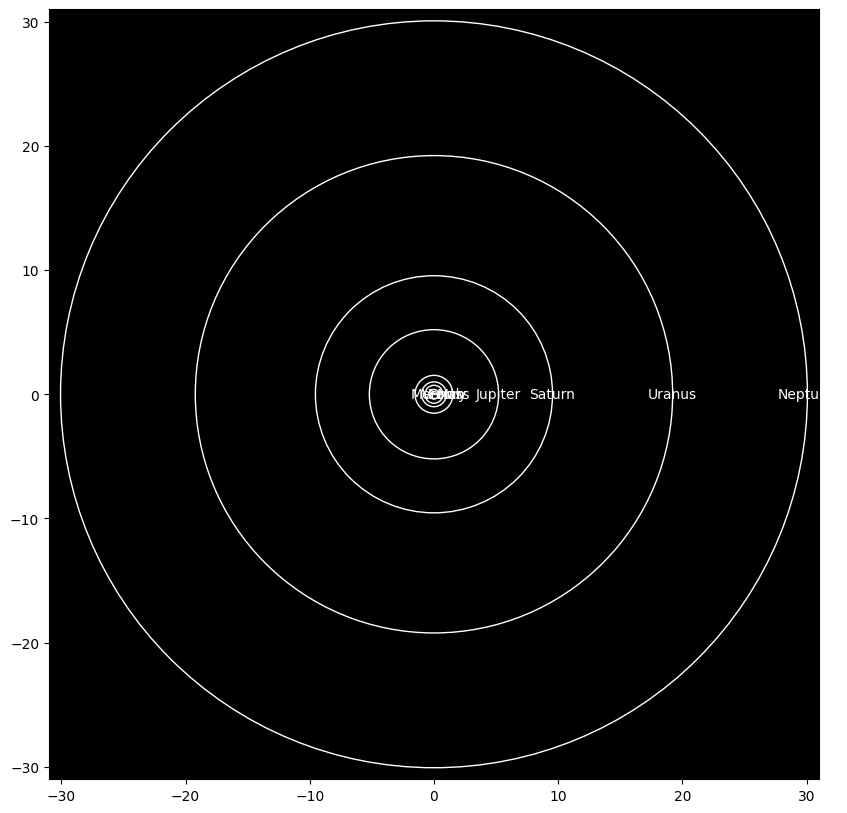
Excel files#
Look at the Budget.xlsx file in the data folder to justify the choice of import below.
Should this be adjusted?
Budget = pd.read_excel('../../data/Budget.xlsx', sheet_name='2019', skiprows=2, index_col=0)
Budget
1 | 2 | 3 | 4 | 5 | 6 | 7 | 8 | 9 | 10 | 11 | 12 | Unnamed: 13 | |
---|---|---|---|---|---|---|---|---|---|---|---|---|---|
SalespersonID | |||||||||||||
102 | NaN | NaN | 54.728491 | 8.053198e+02 | 1010.005526 | NaN | 1572.864176 | 196.077202 | 1896.777586 | 1430.463445 | 347.092894 | 723.918675 | 8.037248e+03 |
125 | 9016.031000 | 12635.710568 | 3975.959267 | 3.541869e+04 | 67254.905958 | 108270.212920 | 129885.185350 | 51801.187615 | 80833.313215 | 14351.065997 | 99034.421805 | 5946.076427 | 6.184228e+05 |
144 | 9870.923721 | 8537.674024 | 34467.719310 | 1.314835e+05 | 63478.807310 | 80332.088219 | 8950.845553 | 51091.397573 | 28463.939431 | 136104.694978 | 29076.741297 | 35033.416700 | 6.168917e+05 |
183 | 1394.216707 | 6598.171435 | 15397.847623 | 2.877323e+04 | 6530.927321 | 158828.572729 | 66426.139653 | 29403.035538 | 139983.518128 | 231592.365267 | 20132.676098 | 17248.364886 | 7.223091e+05 |
194 | NaN | NaN | NaN | NaN | NaN | NaN | NaN | NaN | NaN | NaN | NaN | NaN | NaN |
196 | 2270.020511 | 12633.225198 | 7889.580041 | 2.465794e+03 | 199521.467124 | 6375.290655 | 24857.201539 | 193086.944649 | 66161.225155 | 225389.414595 | 53963.213693 | 37316.866234 | 8.319302e+05 |
215 | 5347.775816 | 98045.092946 | 14818.451817 | 5.493729e+05 | 323788.746583 | 122600.920552 | 79347.415036 | 353588.777730 | 37104.061797 | 41614.097652 | 233643.059453 | 583835.349542 | 2.443107e+06 |
265 | 1995.803045 | 2903.113376 | 16911.287170 | 2.858913e+05 | 143236.464661 | 14227.073545 | 403624.400847 | 76606.898096 | 11670.773263 | 153295.074262 | 202455.468961 | 41727.065582 | 1.354545e+06 |
285 | NaN | NaN | NaN | NaN | NaN | NaN | NaN | NaN | NaN | NaN | NaN | NaN | NaN |
326 | NaN | NaN | NaN | NaN | NaN | NaN | NaN | NaN | NaN | NaN | NaN | NaN | NaN |
660 | NaN | NaN | NaN | NaN | NaN | NaN | NaN | NaN | NaN | NaN | NaN | NaN | NaN |
669 | NaN | NaN | NaN | NaN | NaN | 4962.708683 | 4752.446948 | 11980.303433 | 36185.827317 | 28779.246362 | 52581.712055 | 29698.142511 | 1.689404e+05 |
Grand Total | 29894.770800 | 141352.987547 | 93515.573718 | 1.034211e+06 | 804821.324483 | 495596.867303 | 719416.499101 | 767754.621836 | 402299.435893 | 832556.422558 | 691234.386255 | 751529.200557 | 6.764183e+06 |
# Remove redundant row and column (sums)
Budget = Budget.iloc[:12, :12]
# Exchange NaN values with 0
Budget.replace(np.NAN, 0, inplace=True)
Budget
1 | 2 | 3 | 4 | 5 | 6 | 7 | 8 | 9 | 10 | 11 | 12 | |
---|---|---|---|---|---|---|---|---|---|---|---|---|
SalespersonID | ||||||||||||
102 | 0.000000 | 0.000000 | 54.728491 | 805.319842 | 1010.005526 | 0.000000 | 1572.864176 | 196.077202 | 1896.777586 | 1430.463445 | 347.092894 | 723.918675 |
125 | 9016.031000 | 12635.710568 | 3975.959267 | 35418.687607 | 67254.905958 | 108270.212920 | 129885.185350 | 51801.187615 | 80833.313215 | 14351.065997 | 99034.421805 | 5946.076427 |
144 | 9870.923721 | 8537.674024 | 34467.719310 | 131483.460122 | 63478.807310 | 80332.088219 | 8950.845553 | 51091.397573 | 28463.939431 | 136104.694978 | 29076.741297 | 35033.416700 |
183 | 1394.216707 | 6598.171435 | 15397.847623 | 28773.228192 | 6530.927321 | 158828.572729 | 66426.139653 | 29403.035538 | 139983.518128 | 231592.365267 | 20132.676098 | 17248.364886 |
194 | 0.000000 | 0.000000 | 0.000000 | 0.000000 | 0.000000 | 0.000000 | 0.000000 | 0.000000 | 0.000000 | 0.000000 | 0.000000 | 0.000000 |
196 | 2270.020511 | 12633.225198 | 7889.580041 | 2465.793773 | 199521.467124 | 6375.290655 | 24857.201539 | 193086.944649 | 66161.225155 | 225389.414595 | 53963.213693 | 37316.866234 |
215 | 5347.775816 | 98045.092946 | 14818.451817 | 549372.866029 | 323788.746583 | 122600.920552 | 79347.415036 | 353588.777730 | 37104.061797 | 41614.097652 | 233643.059453 | 583835.349542 |
265 | 1995.803045 | 2903.113376 | 16911.287170 | 285891.263738 | 143236.464661 | 14227.073545 | 403624.400847 | 76606.898096 | 11670.773263 | 153295.074262 | 202455.468961 | 41727.065582 |
285 | 0.000000 | 0.000000 | 0.000000 | 0.000000 | 0.000000 | 0.000000 | 0.000000 | 0.000000 | 0.000000 | 0.000000 | 0.000000 | 0.000000 |
326 | 0.000000 | 0.000000 | 0.000000 | 0.000000 | 0.000000 | 0.000000 | 0.000000 | 0.000000 | 0.000000 | 0.000000 | 0.000000 | 0.000000 |
660 | 0.000000 | 0.000000 | 0.000000 | 0.000000 | 0.000000 | 0.000000 | 0.000000 | 0.000000 | 0.000000 | 0.000000 | 0.000000 | 0.000000 |
669 | 0.000000 | 0.000000 | 0.000000 | 0.000000 | 0.000000 | 4962.708683 | 4752.446948 | 11980.303433 | 36185.827317 | 28779.246362 | 52581.712055 | 29698.142511 |
# Plot monthly budget as boxplot
Budget.boxplot()
plt.show()
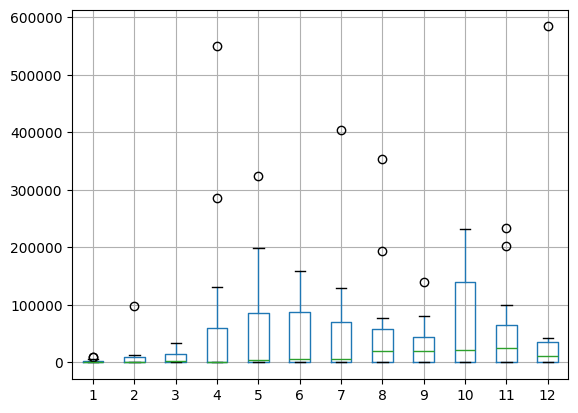
Pandas plotting with different backend#
It is possible to exchange the matplotlib backend in Pandas with something else, e.g., the more interactive Plotly. This may not be a full replacement.
pd.options.plotting.backend = "plotly"
fig = Budget.boxplot()
fig.show() # Exchanged plt.show() with fig.show() to avoid mixing matplotlib and plotly
Other proprietary formats#
Many formats are vendor specific and only accessible because of backward engineering or similar. These are just a handful of such examples:
Some chemical analytical instruments, e.g., Bruker Opus format, Perkin Elmer IR
Media files#
Most image formats and many video formats are more or less directly readable using the Pillow or or PIL libraries.
Medical imaging formats have some Python support, e.g., the DICOM format
Sound files can be opened using pySoundFile, librosa or similar.
JSON - JavaScript Object Notation#
Originally part of the 1999 JavaScript standard.
Popular format for data storage and transfer, supported by a large range of programming languages.
Text-based with fixed formatting but highly flexible structure and contents.
-
a string has limitations/functionality with regard to backslashes , otherwise plain text.
a number is limited to integers, decimal numbers and scientific (float) numbers.
a value can be a string, number, object, array, true, false, or null.
an object is enclosed by curly brackets {} and contains (comma separated) string : value pairs.
an array is enclosed by brackets [] and contains (comma separated) values.
The JSON object is an unordered set.
Nesting is frequently used.
# Simplest? JSON object
import json
my_string = "banana"
my_string_as_json = json.dumps(my_string)
my_string_as_json
'"banana"'
# Check validity of JSON object (exception thrown if not valid)
json.loads(my_string_as_json)
'banana'
# An object, quite similar to a Python dictionary
my_object = {"fruit" : "banana", "color" : "yellow" }
my_object_as_json = json.dumps(my_object)
my_object_as_json
'{"fruit": "banana", "color": "yellow"}'
# Check validity of JSON object (exception thrown if not valid)
json.loads(my_object_as_json)
{'fruit': 'banana', 'color': 'yellow'}
my_object_as_dict = json.loads(my_object_as_json)
my_object_as_dict.keys()
dict_keys(['fruit', 'color'])
JSON variants#
Though JSON itself is highly flexible, standardisation can ease implementation and transferability.
JSON-stat is a format adoptet by, e.g., Statistics Norway and Eurostat.
Standard libraries for handling data.
Especially useful for tabular data.
json-stat2 is the newest standard as of 2023.
# Read text file containing JSON object
import json
with open('../../data/traffic_accidents.txt') as f:
json_string = f.read()
json_object = json.loads(json_string)
json_object
{'class': 'dataset',
'label': '06794: Persons killed or injured in road traffic accidents, by degree of damage, sex, group of road user, contents and month',
'source': 'Statistics Norway',
'updated': '2023-08-18T06:00:00Z',
'id': ['Skadegrad', 'Kjonn', 'Trafikkantgruppe', 'ContentsCode', 'Tid'],
'size': [6, 2, 8, 1, 295],
'dimension': {'Skadegrad': {'extension': {'show': 'value'},
'label': 'degree of damage',
'category': {'index': {'01': 0,
'20': 1,
'02': 2,
'03': 3,
'04': 4,
'05': 5},
'label': {'01': 'Killed',
'20': 'All injured',
'02': 'Dangerously injured',
'03': 'Seriously injured',
'04': 'Slightly injured',
'05': 'Degree of injury unspecified'}}},
'Kjonn': {'extension': {'show': 'value'},
'label': 'sex',
'category': {'index': {'1': 0, '2': 1},
'label': {'1': 'Males', '2': 'Females'}},
'link': {'describedby': [{'extension': {'Kjonn': 'urn:ssb:classification:klass:2'}}]}},
'Trafikkantgruppe': {'extension': {'show': 'value'},
'label': 'group of road user',
'category': {'index': {'1': 0,
'2': 1,
'3': 2,
'4': 3,
'5': 4,
'6': 5,
'7': 6,
'8': 7},
'label': {'1': 'Drivers of car',
'2': 'Passengers of car',
'3': 'Drivers and passengers on motorcycle',
'4': 'Drivers and passengers on moped',
'5': 'Drivers and passengers on cycle',
'6': 'Pedestrians',
'7': 'Persons sledging',
'8': 'Others'}}},
'ContentsCode': {'extension': {'show': 'value'},
'label': 'contents',
'category': {'index': {'SkaddDrept': 0},
'label': {'SkaddDrept': 'Persons killed or injured'},
'unit': {'SkaddDrept': {'base': 'persons', 'decimals': 0}}}},
'Tid': {'extension': {'show': 'code'},
'label': 'month',
'category': {'index': {'1999M01': 0,
'1999M02': 1,
'1999M03': 2,
'1999M04': 3,
'1999M05': 4,
'1999M06': 5,
'1999M07': 6,
'1999M08': 7,
'1999M09': 8,
'1999M10': 9,
'1999M11': 10,
'1999M12': 11,
'2000M01': 12,
'2000M02': 13,
'2000M03': 14,
'2000M04': 15,
'2000M05': 16,
'2000M06': 17,
'2000M07': 18,
'2000M08': 19,
'2000M09': 20,
'2000M10': 21,
'2000M11': 22,
'2000M12': 23,
'2001M01': 24,
'2001M02': 25,
'2001M03': 26,
'2001M04': 27,
'2001M05': 28,
'2001M06': 29,
'2001M07': 30,
'2001M08': 31,
'2001M09': 32,
'2001M10': 33,
'2001M11': 34,
'2001M12': 35,
'2002M01': 36,
'2002M02': 37,
'2002M03': 38,
'2002M04': 39,
'2002M05': 40,
'2002M06': 41,
'2002M07': 42,
'2002M08': 43,
'2002M09': 44,
'2002M10': 45,
'2002M11': 46,
'2002M12': 47,
'2003M01': 48,
'2003M02': 49,
'2003M03': 50,
'2003M04': 51,
'2003M05': 52,
'2003M06': 53,
'2003M07': 54,
'2003M08': 55,
'2003M09': 56,
'2003M10': 57,
'2003M11': 58,
'2003M12': 59,
'2004M01': 60,
'2004M02': 61,
'2004M03': 62,
'2004M04': 63,
'2004M05': 64,
'2004M06': 65,
'2004M07': 66,
'2004M08': 67,
'2004M09': 68,
'2004M10': 69,
'2004M11': 70,
'2004M12': 71,
'2005M01': 72,
'2005M02': 73,
'2005M03': 74,
'2005M04': 75,
'2005M05': 76,
'2005M06': 77,
'2005M07': 78,
'2005M08': 79,
'2005M09': 80,
'2005M10': 81,
'2005M11': 82,
'2005M12': 83,
'2006M01': 84,
'2006M02': 85,
'2006M03': 86,
'2006M04': 87,
'2006M05': 88,
'2006M06': 89,
'2006M07': 90,
'2006M08': 91,
'2006M09': 92,
'2006M10': 93,
'2006M11': 94,
'2006M12': 95,
'2007M01': 96,
'2007M02': 97,
'2007M03': 98,
'2007M04': 99,
'2007M05': 100,
'2007M06': 101,
'2007M07': 102,
'2007M08': 103,
'2007M09': 104,
'2007M10': 105,
'2007M11': 106,
'2007M12': 107,
'2008M01': 108,
'2008M02': 109,
'2008M03': 110,
'2008M04': 111,
'2008M05': 112,
'2008M06': 113,
'2008M07': 114,
'2008M08': 115,
'2008M09': 116,
'2008M10': 117,
'2008M11': 118,
'2008M12': 119,
'2009M01': 120,
'2009M02': 121,
'2009M03': 122,
'2009M04': 123,
'2009M05': 124,
'2009M06': 125,
'2009M07': 126,
'2009M08': 127,
'2009M09': 128,
'2009M10': 129,
'2009M11': 130,
'2009M12': 131,
'2010M01': 132,
'2010M02': 133,
'2010M03': 134,
'2010M04': 135,
'2010M05': 136,
'2010M06': 137,
'2010M07': 138,
'2010M08': 139,
'2010M09': 140,
'2010M10': 141,
'2010M11': 142,
'2010M12': 143,
'2011M01': 144,
'2011M02': 145,
'2011M03': 146,
'2011M04': 147,
'2011M05': 148,
'2011M06': 149,
'2011M07': 150,
'2011M08': 151,
'2011M09': 152,
'2011M10': 153,
'2011M11': 154,
'2011M12': 155,
'2012M01': 156,
'2012M02': 157,
'2012M03': 158,
'2012M04': 159,
'2012M05': 160,
'2012M06': 161,
'2012M07': 162,
'2012M08': 163,
'2012M09': 164,
'2012M10': 165,
'2012M11': 166,
'2012M12': 167,
'2013M01': 168,
'2013M02': 169,
'2013M03': 170,
'2013M04': 171,
'2013M05': 172,
'2013M06': 173,
'2013M07': 174,
'2013M08': 175,
'2013M09': 176,
'2013M10': 177,
'2013M11': 178,
'2013M12': 179,
'2014M01': 180,
'2014M02': 181,
'2014M03': 182,
'2014M04': 183,
'2014M05': 184,
'2014M06': 185,
'2014M07': 186,
'2014M08': 187,
'2014M09': 188,
'2014M10': 189,
'2014M11': 190,
'2014M12': 191,
'2015M01': 192,
'2015M02': 193,
'2015M03': 194,
'2015M04': 195,
'2015M05': 196,
'2015M06': 197,
'2015M07': 198,
'2015M08': 199,
'2015M09': 200,
'2015M10': 201,
'2015M11': 202,
'2015M12': 203,
'2016M01': 204,
'2016M02': 205,
'2016M03': 206,
'2016M04': 207,
'2016M05': 208,
'2016M06': 209,
'2016M07': 210,
'2016M08': 211,
'2016M09': 212,
'2016M10': 213,
'2016M11': 214,
'2016M12': 215,
'2017M01': 216,
'2017M02': 217,
'2017M03': 218,
'2017M04': 219,
'2017M05': 220,
'2017M06': 221,
'2017M07': 222,
'2017M08': 223,
'2017M09': 224,
'2017M10': 225,
'2017M11': 226,
'2017M12': 227,
'2018M01': 228,
'2018M02': 229,
'2018M03': 230,
'2018M04': 231,
'2018M05': 232,
'2018M06': 233,
'2018M07': 234,
'2018M08': 235,
'2018M09': 236,
'2018M10': 237,
'2018M11': 238,
'2018M12': 239,
'2019M01': 240,
'2019M02': 241,
'2019M03': 242,
'2019M04': 243,
'2019M05': 244,
'2019M06': 245,
'2019M07': 246,
'2019M08': 247,
'2019M09': 248,
'2019M10': 249,
'2019M11': 250,
'2019M12': 251,
'2020M01': 252,
'2020M02': 253,
'2020M03': 254,
'2020M04': 255,
'2020M05': 256,
'2020M06': 257,
'2020M07': 258,
'2020M08': 259,
'2020M09': 260,
'2020M10': 261,
'2020M11': 262,
'2020M12': 263,
'2021M01': 264,
'2021M02': 265,
'2021M03': 266,
'2021M04': 267,
'2021M05': 268,
'2021M06': 269,
'2021M07': 270,
'2021M08': 271,
'2021M09': 272,
'2021M10': 273,
'2021M11': 274,
'2021M12': 275,
'2022M01': 276,
'2022M02': 277,
'2022M03': 278,
'2022M04': 279,
'2022M05': 280,
'2022M06': 281,
'2022M07': 282,
'2022M08': 283,
'2022M09': 284,
'2022M10': 285,
'2022M11': 286,
'2022M12': 287,
'2023M01': 288,
'2023M02': 289,
'2023M03': 290,
'2023M04': 291,
'2023M05': 292,
'2023M06': 293,
'2023M07': 294},
'label': {'1999M01': '1999M01',
'1999M02': '1999M02',
'1999M03': '1999M03',
'1999M04': '1999M04',
'1999M05': '1999M05',
'1999M06': '1999M06',
'1999M07': '1999M07',
'1999M08': '1999M08',
'1999M09': '1999M09',
'1999M10': '1999M10',
'1999M11': '1999M11',
'1999M12': '1999M12',
'2000M01': '2000M01',
'2000M02': '2000M02',
'2000M03': '2000M03',
'2000M04': '2000M04',
'2000M05': '2000M05',
'2000M06': '2000M06',
'2000M07': '2000M07',
'2000M08': '2000M08',
'2000M09': '2000M09',
'2000M10': '2000M10',
'2000M11': '2000M11',
'2000M12': '2000M12',
'2001M01': '2001M01',
'2001M02': '2001M02',
'2001M03': '2001M03',
'2001M04': '2001M04',
'2001M05': '2001M05',
'2001M06': '2001M06',
'2001M07': '2001M07',
'2001M08': '2001M08',
'2001M09': '2001M09',
'2001M10': '2001M10',
'2001M11': '2001M11',
'2001M12': '2001M12',
'2002M01': '2002M01',
'2002M02': '2002M02',
'2002M03': '2002M03',
'2002M04': '2002M04',
'2002M05': '2002M05',
'2002M06': '2002M06',
'2002M07': '2002M07',
'2002M08': '2002M08',
'2002M09': '2002M09',
'2002M10': '2002M10',
'2002M11': '2002M11',
'2002M12': '2002M12',
'2003M01': '2003M01',
'2003M02': '2003M02',
'2003M03': '2003M03',
'2003M04': '2003M04',
'2003M05': '2003M05',
'2003M06': '2003M06',
'2003M07': '2003M07',
'2003M08': '2003M08',
'2003M09': '2003M09',
'2003M10': '2003M10',
'2003M11': '2003M11',
'2003M12': '2003M12',
'2004M01': '2004M01',
'2004M02': '2004M02',
'2004M03': '2004M03',
'2004M04': '2004M04',
'2004M05': '2004M05',
'2004M06': '2004M06',
'2004M07': '2004M07',
'2004M08': '2004M08',
'2004M09': '2004M09',
'2004M10': '2004M10',
'2004M11': '2004M11',
'2004M12': '2004M12',
'2005M01': '2005M01',
'2005M02': '2005M02',
'2005M03': '2005M03',
'2005M04': '2005M04',
'2005M05': '2005M05',
'2005M06': '2005M06',
'2005M07': '2005M07',
'2005M08': '2005M08',
'2005M09': '2005M09',
'2005M10': '2005M10',
'2005M11': '2005M11',
'2005M12': '2005M12',
'2006M01': '2006M01',
'2006M02': '2006M02',
'2006M03': '2006M03',
'2006M04': '2006M04',
'2006M05': '2006M05',
'2006M06': '2006M06',
'2006M07': '2006M07',
'2006M08': '2006M08',
'2006M09': '2006M09',
'2006M10': '2006M10',
'2006M11': '2006M11',
'2006M12': '2006M12',
'2007M01': '2007M01',
'2007M02': '2007M02',
'2007M03': '2007M03',
'2007M04': '2007M04',
'2007M05': '2007M05',
'2007M06': '2007M06',
'2007M07': '2007M07',
'2007M08': '2007M08',
'2007M09': '2007M09',
'2007M10': '2007M10',
'2007M11': '2007M11',
'2007M12': '2007M12',
'2008M01': '2008M01',
'2008M02': '2008M02',
'2008M03': '2008M03',
'2008M04': '2008M04',
'2008M05': '2008M05',
'2008M06': '2008M06',
'2008M07': '2008M07',
'2008M08': '2008M08',
'2008M09': '2008M09',
'2008M10': '2008M10',
'2008M11': '2008M11',
'2008M12': '2008M12',
'2009M01': '2009M01',
'2009M02': '2009M02',
'2009M03': '2009M03',
'2009M04': '2009M04',
'2009M05': '2009M05',
'2009M06': '2009M06',
'2009M07': '2009M07',
'2009M08': '2009M08',
'2009M09': '2009M09',
'2009M10': '2009M10',
'2009M11': '2009M11',
'2009M12': '2009M12',
'2010M01': '2010M01',
'2010M02': '2010M02',
'2010M03': '2010M03',
'2010M04': '2010M04',
'2010M05': '2010M05',
'2010M06': '2010M06',
'2010M07': '2010M07',
'2010M08': '2010M08',
'2010M09': '2010M09',
'2010M10': '2010M10',
'2010M11': '2010M11',
'2010M12': '2010M12',
'2011M01': '2011M01',
'2011M02': '2011M02',
'2011M03': '2011M03',
'2011M04': '2011M04',
'2011M05': '2011M05',
'2011M06': '2011M06',
'2011M07': '2011M07',
'2011M08': '2011M08',
'2011M09': '2011M09',
'2011M10': '2011M10',
'2011M11': '2011M11',
'2011M12': '2011M12',
'2012M01': '2012M01',
'2012M02': '2012M02',
'2012M03': '2012M03',
'2012M04': '2012M04',
'2012M05': '2012M05',
'2012M06': '2012M06',
'2012M07': '2012M07',
'2012M08': '2012M08',
'2012M09': '2012M09',
'2012M10': '2012M10',
'2012M11': '2012M11',
'2012M12': '2012M12',
'2013M01': '2013M01',
'2013M02': '2013M02',
'2013M03': '2013M03',
'2013M04': '2013M04',
'2013M05': '2013M05',
'2013M06': '2013M06',
'2013M07': '2013M07',
'2013M08': '2013M08',
'2013M09': '2013M09',
'2013M10': '2013M10',
'2013M11': '2013M11',
'2013M12': '2013M12',
'2014M01': '2014M01',
'2014M02': '2014M02',
'2014M03': '2014M03',
'2014M04': '2014M04',
'2014M05': '2014M05',
'2014M06': '2014M06',
'2014M07': '2014M07',
'2014M08': '2014M08',
'2014M09': '2014M09',
'2014M10': '2014M10',
'2014M11': '2014M11',
'2014M12': '2014M12',
'2015M01': '2015M01',
'2015M02': '2015M02',
'2015M03': '2015M03',
'2015M04': '2015M04',
'2015M05': '2015M05',
'2015M06': '2015M06',
'2015M07': '2015M07',
'2015M08': '2015M08',
'2015M09': '2015M09',
'2015M10': '2015M10',
'2015M11': '2015M11',
'2015M12': '2015M12',
'2016M01': '2016M01',
'2016M02': '2016M02',
'2016M03': '2016M03',
'2016M04': '2016M04',
'2016M05': '2016M05',
'2016M06': '2016M06',
'2016M07': '2016M07',
'2016M08': '2016M08',
'2016M09': '2016M09',
'2016M10': '2016M10',
'2016M11': '2016M11',
'2016M12': '2016M12',
'2017M01': '2017M01',
'2017M02': '2017M02',
'2017M03': '2017M03',
'2017M04': '2017M04',
'2017M05': '2017M05',
'2017M06': '2017M06',
'2017M07': '2017M07',
'2017M08': '2017M08',
'2017M09': '2017M09',
'2017M10': '2017M10',
'2017M11': '2017M11',
'2017M12': '2017M12',
'2018M01': '2018M01',
'2018M02': '2018M02',
'2018M03': '2018M03',
'2018M04': '2018M04',
'2018M05': '2018M05',
'2018M06': '2018M06',
'2018M07': '2018M07',
'2018M08': '2018M08',
'2018M09': '2018M09',
'2018M10': '2018M10',
'2018M11': '2018M11',
'2018M12': '2018M12',
'2019M01': '2019M01',
'2019M02': '2019M02',
'2019M03': '2019M03',
'2019M04': '2019M04',
'2019M05': '2019M05',
'2019M06': '2019M06',
'2019M07': '2019M07',
'2019M08': '2019M08',
'2019M09': '2019M09',
'2019M10': '2019M10',
'2019M11': '2019M11',
'2019M12': '2019M12',
'2020M01': '2020M01',
'2020M02': '2020M02',
'2020M03': '2020M03',
'2020M04': '2020M04',
'2020M05': '2020M05',
'2020M06': '2020M06',
'2020M07': '2020M07',
'2020M08': '2020M08',
'2020M09': '2020M09',
'2020M10': '2020M10',
'2020M11': '2020M11',
'2020M12': '2020M12',
'2021M01': '2021M01',
'2021M02': '2021M02',
'2021M03': '2021M03',
'2021M04': '2021M04',
'2021M05': '2021M05',
'2021M06': '2021M06',
'2021M07': '2021M07',
'2021M08': '2021M08',
'2021M09': '2021M09',
'2021M10': '2021M10',
'2021M11': '2021M11',
'2021M12': '2021M12',
'2022M01': '2022M01',
'2022M02': '2022M02',
'2022M03': '2022M03',
'2022M04': '2022M04',
'2022M05': '2022M05',
'2022M06': '2022M06',
'2022M07': '2022M07',
'2022M08': '2022M08',
'2022M09': '2022M09',
'2022M10': '2022M10',
'2022M11': '2022M11',
'2022M12': '2022M12',
'2023M01': '2023M01',
'2023M02': '2023M02',
'2023M03': '2023M03',
'2023M04': '2023M04',
'2023M05': '2023M05',
'2023M06': '2023M06',
'2023M07': '2023M07'}}}},
'value': [8,
6,
5,
9,
12,
12,
7,
11,
9,
7,
13,
16,
9,
5,
9,
7,
13,
10,
11,
12,
16,
8,
11,
8,
16,
7,
7,
10,
10,
9,
11,
6,
11,
5,
8,
7,
13,
9,
7,
8,
15,
12,
5,
11,
6,
15,
7,
12,
7,
4,
10,
10,
12,
5,
15,
10,
9,
9,
14,
8,
6,
11,
7,
5,
6,
9,
7,
4,
9,
13,
8,
13,
8,
7,
7,
3,
5,
7,
13,
5,
5,
5,
11,
6,
7,
8,
7,
4,
11,
5,
10,
3,
11,
6,
17,
11,
10,
3,
2,
5,
6,
7,
2,
10,
8,
14,
7,
7,
10,
9,
10,
7,
12,
8,
6,
14,
4,
12,
3,
8,
7,
4,
4,
7,
6,
6,
8,
10,
10,
4,
7,
10,
9,
6,
5,
6,
5,
14,
10,
10,
11,
7,
5,
5,
5,
4,
6,
4,
5,
7,
7,
5,
8,
4,
6,
6,
4,
7,
5,
4,
3,
1,
4,
6,
4,
4,
3,
0,
6,
3,
7,
6,
3,
8,
7,
9,
5,
8,
3,
9,
2,
6,
3,
0,
10,
7,
6,
2,
5,
2,
4,
3,
4,
3,
8,
2,
2,
0,
2,
3,
7,
4,
5,
6,
5,
7,
4,
1,
1,
5,
8,
2,
3,
3,
4,
2,
3,
1,
3,
3,
5,
1,
2,
4,
2,
2,
7,
6,
4,
5,
1,
5,
5,
4,
4,
2,
3,
7,
10,
3,
3,
1,
6,
4,
4,
5,
1,
3,
4,
5,
4,
4,
5,
6,
4,
2,
2,
2,
2,
4,
1,
2,
3,
4,
2,
1,
1,
0,
4,
3,
3,
4,
4,
2,
2,
3,
0,
1,
3,
5,
3,
3,
5,
5,
0,
3,
5,
3,
4,
3,
3,
4,
5,
3,
7,
2,
1,
0,
2,
4,
7,
6,
1,
1,
8,
2,
2,
4,
2,
2,
3,
3,
5,
8,
6,
9,
2,
2,
3,
1,
2,
3,
4,
2,
1,
2,
2,
2,
0,
4,
1,
5,
2,
1,
2,
6,
2,
6,
5,
3,
4,
1,
3,
0,
4,
2,
2,
3,
2,
1,
5,
4,
2,
5,
2,
2,
3,
1,
4,
2,
5,
3,
1,
0,
3,
0,
3,
3,
1,
1,
2,
0,
3,
1,
4,
2,
0,
2,
2,
1,
5,
0,
0,
1,
1,
3,
0,
1,
3,
1,
1,
4,
4,
2,
1,
1,
1,
2,
3,
3,
1,
3,
1,
4,
2,
3,
2,
4,
1,
2,
1,
0,
2,
1,
5,
5,
0,
1,
2,
2,
4,
2,
1,
6,
2,
1,
3,
1,
1,
2,
4,
2,
3,
1,
4,
1,
2,
0,
0,
3,
1,
1,
0,
0,
3,
1,
1,
0,
2,
1,
3,
3,
2,
0,
0,
2,
0,
2,
1,
1,
0,
0,
1,
1,
1,
0,
0,
0,
2,
1,
2,
1,
1,
2,
3,
2,
2,
0,
0,
1,
1,
3,
3,
3,
0,
0,
0,
0,
0,
0,
0,
0,
0,
1,
1,
0,
1,
2,
0,
2,
2,
0,
2,
1,
0,
3,
0,
0,
1,
1,
0,
0,
0,
0,
0,
0,
1,
0,
0,
0,
0,
0,
1,
0,
0,
0,
0,
0,
0,
0,
0,
3,
0,
0,
0,
1,
1,
0,
1,
0,
0,
2,
1,
0,
2,
1,
0,
1,
0,
1,
0,
1,
0,
0,
2,
0,
1,
0,
0,
1,
0,
0,
1,
3,
1,
0,
2,
0,
1,
0,
0,
0,
0,
0,
0,
5,
1,
1,
0,
0,
0,
0,
0,
0,
0,
1,
2,
1,
1,
1,
0,
0,
1,
1,
5,
6,
5,
4,
5,
1,
1,
0,
0,
0,
1,
3,
4,
11,
7,
4,
4,
1,
3,
0,
0,
0,
0,
1,
3,
8,
7,
7,
1,
0,
0,
0,
1,
0,
2,
0,
6,
4,
6,
0,
11,
3,
0,
0,
0,
1,
0,
2,
5,
5,
6,
4,
2,
1,
0,
0,
0,
0,
1,
5,
2,
6,
7,
7,
4,
0,
0,
0,
1,
1,
3,
2,
3,
2,
3,
5,
3,
2,
2,
0,
0,
0,
0,
0,
4,
7,
5,
6,
3,
2,
0,
0,
0,
0,
0,
2,
2,
3,
6,
6,
6,
1,
0,
1,
0,
0,
0,
2,
1,
9,
6,
6,
3,
1,
0,
0,
0,
0,
1,
3,
5,
5,
4,
5,
4,
0,
0,
0,
0,
0,
0,
1,
1,
3,
10,
5,
1,
2,
0,
0,
0,
1,
1,
1,
1,
4,
2,
2,
0,
1,
0,
0,
0,
0,
0,
0,
3,
3,
3,
4,
0,
3,
0,
0,
0,
1,
0,
0,
3,
0,
3,
6,
5,
1,
0,
0,
0,
0,
0,
3,
3,
2,
5,
3,
1,
0,
0,
0,
0,
0,
0,
2,
4,
5,
3,
2,
1,
0,
0,
0,
0,
0,
0,
3,
1,
6,
3,
3,
1,
5,
0,
0,
0,
0,
0,
0,
4,
4,
2,
3,
0,
2,
0,
0,
0,
0,
1,
0,
1,
2,
2,
3,
3,
0,
0,
0,
0,
0,
0,
3,
1,
5,
1,
1,
3,
1,
0,
0,
0,
0,
0,
1,
3,
6,
2,
3,
0,
0,
0,
0,
0,
0,
0,
1,
1,
1,
2,
3,
5,
1,
1,
0,
0,
1,
0,
1,
5,
6,
3,
2,
2,
0,
0,
0,
0,
0,
0,
2,
3,
1,
1,
0,
0,
1,
2,
2,
0,
0,
0,
0,
0,
0,
0,
0,
0,
0,
1,
0,
1,
1,
0,
0,
0,
1,
1,
0,
0,
0,
0,
0,
1,
0,
2,
2,
0,
0,
0,
0,
0,
0,
1,
1,
1,
1,
1,
0,
0,
1,
0,
0,
0,
1,
1,
1,
0,
0,
0,
2,
0,
0,
2,
0,
1,
0,
0,
1,
2,
2,
1,
0,
0,
0,
0,
1,
0,
0,
0,
0,
1,
0,
1,
0,
0,
0,
0,
0,
0,
0,
0,
0,
0,
0,
2,
0,
1,
0,
0,
0,
0,
0,
0,
2,
0,
1,
0,
0,
2,
1,
1,
0,
0,
1,
0,
0,
1,
2,
...],
'role': {'time': ['Tid'], 'metric': ['ContentsCode']},
'version': '2.0',
'extension': {'px': {'infofile': 'None', 'tableid': '06794', 'decimals': 0}}}
# Convert json_string to a JSON-stat object and then to a Pandas dataframe
from pyjstat import pyjstat
dataset = pyjstat.Dataset.read(json_string)
df = dataset.write('dataframe')
print(df.shape)
df.head()
(28320, 6)
degree of damage | sex | group of road user | contents | month | value | |
---|---|---|---|---|---|---|
0 | Killed | Males | Drivers of car | Persons killed or injured | 1999M01 | 8 |
1 | Killed | Males | Drivers of car | Persons killed or injured | 1999M02 | 6 |
2 | Killed | Males | Drivers of car | Persons killed or injured | 1999M03 | 5 |
3 | Killed | Males | Drivers of car | Persons killed or injured | 1999M04 | 9 |
4 | Killed | Males | Drivers of car | Persons killed or injured | 1999M05 | 12 |
Exercise#
We want to make a family tree consisting of child-parent JSON objects.
Create a JSON list which contains two objects of equal structure (i.e., same nesting and names).
Each object should contain a name : value pair with the child name and a list of parents (name : value pairs).
Think:
Are there alternative ways to code this? (hint: unique dictionary keys)
If this JSON object was to be parsed for printing, inclusion in a database or other, are there exceptions one would have to consider?
Extensible Markup Language (XML)#
Defined by the World Wide Web Consortium in 1998 (v1.0); current version from 2006 (v1.1, 2nd edition).
Uses tags like HTML code, but much more flexible.
Hundreds of document formats, several industry data standards and communication protocols, web page formats etc. are based on XML.
As with JSON, there are many standardised formats defined using the base rules of XML.
More overhead in the file format, less readable, needs parser, more standardised, overlapping use in data transfer.
Accepts most of the UTF-8 encoding (see below) including Chinese, Armenian, Cyrillic.
''' A valid XML file
<?xml version="1.0" encoding="UTF-8"?>
<俄语 լեզու="ռուսերեն">данные</俄语>
'''
' A valid XML file\n<?xml version="1.0" encoding="UTF-8"?>\n<俄语 լեզու="ռուսերեն">данные</俄语>\n'
# Equivalent JSON and XML documents
'''
{"guests":[
{ "firstName":"John", "lastName":"Doe" },
{ "firstName":"María", "lastName":"García" },
{ "firstName":"Nikki", "lastName":"Wolf" }
]}
<guests>
<guest>
<firstName>John</firstName> <lastName>Doe</lastName>
</guest>
<guest>
<firstName>María</firstName> <lastName>García</lastName>
</guest>
<guest>
<firstName>Nikki</firstName> <lastName>Wolf</lastName>
</guest>
</guests>
'''
' \n{"guests":[\n { "firstName":"John", "lastName":"Doe" },\n { "firstName":"María", "lastName":"García" },\n { "firstName":"Nikki", "lastName":"Wolf" }\n]}\n\n<guests>\n <guest>\n <firstName>John</firstName> <lastName>Doe</lastName>\n </guest>\n <guest>\n <firstName>María</firstName> <lastName>García</lastName>\n </guest>\n <guest>\n <firstName>Nikki</firstName> <lastName>Wolf</lastName>\n </guest>\n</guests>\n'
Large data#
Real data can be exeedingly large; gigabytes, terabytes, …
Pandas takes you only part of the way.
Modin is an example of a simple Pandas replacement that scales much further (>1TB).
Choose backend: Ray, Dask, Unidist, and use import modin.pandas as pd
PySpark with Apache Spark takes this to a distributed level but also demands more installation and configuration.
We will test Spark in conjunction with a database later.
HDF5#
Data container for large and/or heterogeneous data.
Appears as a single file on a computer.
Can be accessed and browsed without loading/unpacking its contents.
Can group objects together, e.g., measurements and metadata.
Data can be accessed through different views that require different relations or hierachies.
Often used quite simply with data, models, and results in a single file.
Resembles a flexible NumPy array.
Encoding#
Most modern data storage use UTF-8 encoding
Universal Coded Character Set Transformation Format - 8-bit, or just Unicode Transformation Format.
One to four 8-bit code units enabling 1 112 064 character codes.
JSON are among formats one assumes are UTF-8 encoded.
However, there are many standards, so no guarantees are given.
ASCII, WINDOWS-1252 (Nordic), ISO-88xx-y, etc. may need special treatment or translation, especially if different connected apps or services have their own basic assumptions.
Old data files and vendor specific file version are most likely to be problematic.
See also
Resources
YouTube: Learn JSON in 10 minutes (12m:00s)
YouTube: An Introduction to HDF5 (10m:23s) (full course available)
Show code cell content
# Dummy cell to ensure Plotly graphics are shown
import plotly.graph_objects as go
f = go.FigureWidget([go.Scatter(x=[1,1], y=[1,1], mode='markers')])